G2I 2023 Session Recap
AI is hot right now. Why is this suddenly the tech everyone is talking about? For one thing, AI has gotten significantly better, even just in the last year. With this, interest in adding AI to enterprise tech stacks has grown exponentially. But, despite what technologies like ChatGPT might suggest, you can’t just flip the switch and turn on AI if you want to achieve actual value.
This is what Brandon Fischer and Kelly Hopson spoke about at Gateway to Innovation on April 26, 2023. You can view the full recording of the session, but here’s a recap of the four pitfalls to avoid and how to maximize your AI investment.
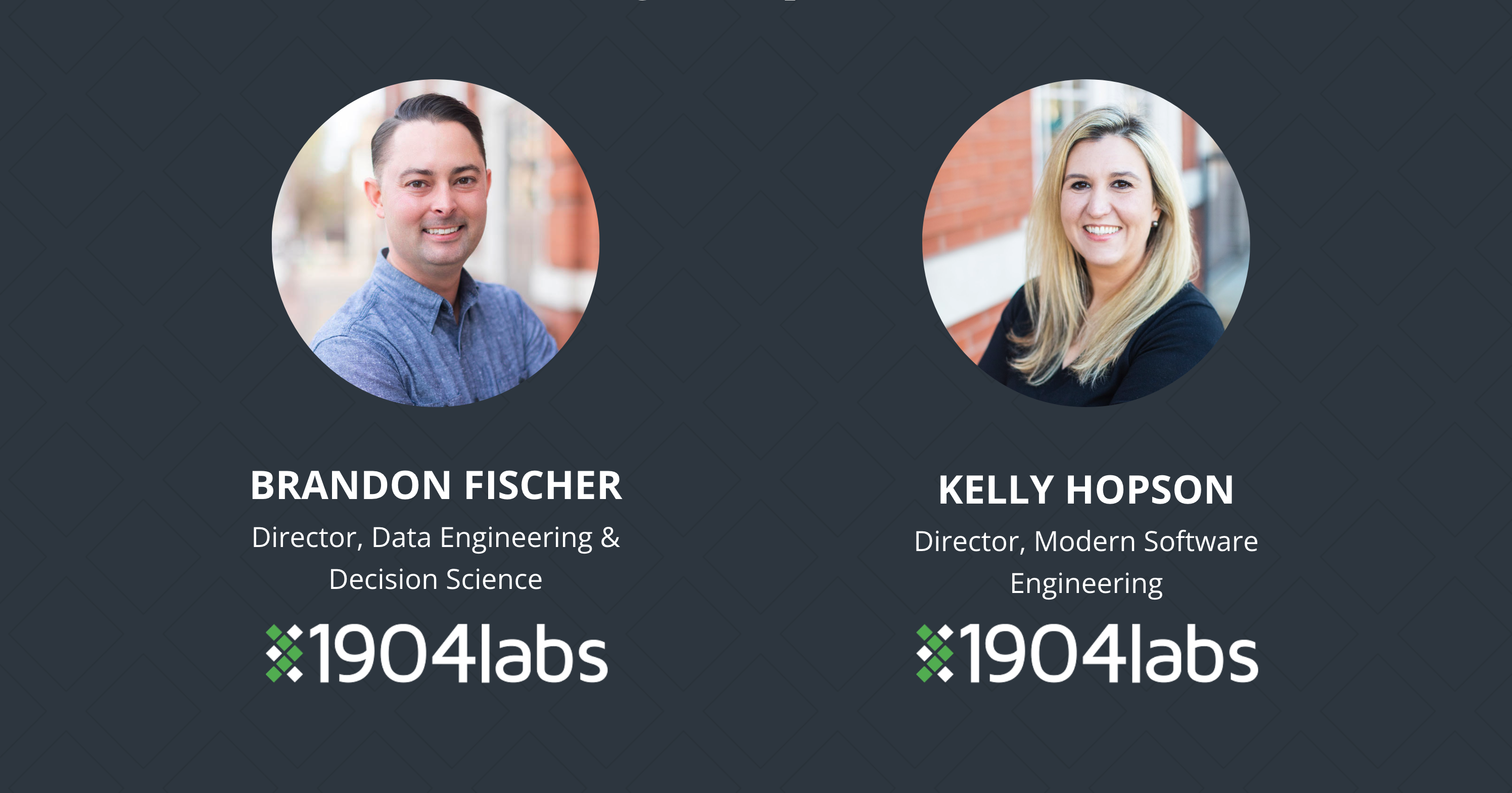
Mistake #1: Pushing for AI because it’s trendy
It’s easy to see a new hot technology that’s trendy and want to jump on board to get ROI. Does this conversation sound familiar?
Business Executive: “I want to add AI to our tech stack.”
IT Leader: “Awesome! What did you have in mind?”
Business Executive: “I want to make sure we stay ahead of the market and innovative - and we need it yesterday.”
Wanting to be cutting edge and ahead of the competition is a valid driving factor. But it can also mean you’re potentially wasting money if there’s no plan in place.
Solution: Start by developing a clear AI strategy
Kelly and Brandon pointed out that there are specific use cases and business objectives that are perfect for AI (and some that are not).
Start by identifying the right problem to solve with AI.
Identify the specific business objectives, use cases, and goals that AI can have an impact on. AI isn’t a magic bullet. For example, ChatGPT isn’t going to be good at detecting fraud. Be realistic about what AI can - and more importantly, can’t - do. So figure out the problem you want to solve first and then pick the model that is best suited to that problem.
Remember that humans will be the one interacting with the AI
Human behavior is hard to change. Implementing an AI system no one wants or needs, will result in something that no one uses and will be a huge waste of time, energy, and money. As part of your AI strategy you will need to think through how humans will interact with the AI. Will they send messages, send images, speak to it? How does it fit with existing processes?
Build vs. Buy vs. Tune
There are many options for achieving AI capabilities, just like any tech solution. You can of course buy or license AI bots from various providers, you can undertake the process of building your own AI system, or you can combine these options and tune an existing AI bot to suit your unique situation.
If you decide to build your own AI bot, know that building and implementing an AI system from scratch takes a lot of time and resources. You will need to collect and curate all of the data, build, test and validate your AI model(s), and establish the underlying infrastructure to deploy and support your system in production. This isn’t meant to scare you off, but building from scratch might only make sense if there isn’t an existing model that can solve your problem. Before you set out on this journey, ensure that your value proposition can offset the cost of building from scratch.
Buying or licensing an AI system can absolutely speed up your time to market. However, before buying anything, ask yourself if the AI system genuinely meets your needs and does what you want it to do. Models you buy may have a black box around it. You are not going to be able to fundamentally change the output of the model (for example, a text model won’t output images), and you may have very little insight into how the model actually works.
Tuning an existing AI system can be a really beneficial middle ground between buy and build. Tuning the AI system allows you to target an existing AI bot at your data set so that its responses are tuned to your problem. Brandon spoke about an example where he and his team used one of the OpenAI models and tuned it by feeding it every Dr. Seuss book. It figured out the style with short rhyming sentences and silly made up words, but the content wasn’t cohesive. The most current versions of all the major large language models are very responsive to tuning. In many cases, tuning may be a great option because it allows you to get to market fairly quickly and will produce results that are more specific to your problem set.
Mistake #2: Underestimating the time & effort
From the business side of the house, it may seem fairly simple and straightforward to add AI/ML into your tech stack. But it’s actually a lot more complicated.
As a technologist, you realize that the technical architecture looks something like the image below. Your business is generating data, but you most likely also get data from external sources. All that data goes through ingestion and ETL processes before it lands in the data store. This data is fed back to the business to drive decision making through an operational data hub and is utilized by an analytics layer for insights and reporting. Before you turn on AI, you need to have all of these different pieces in place to make it effective.
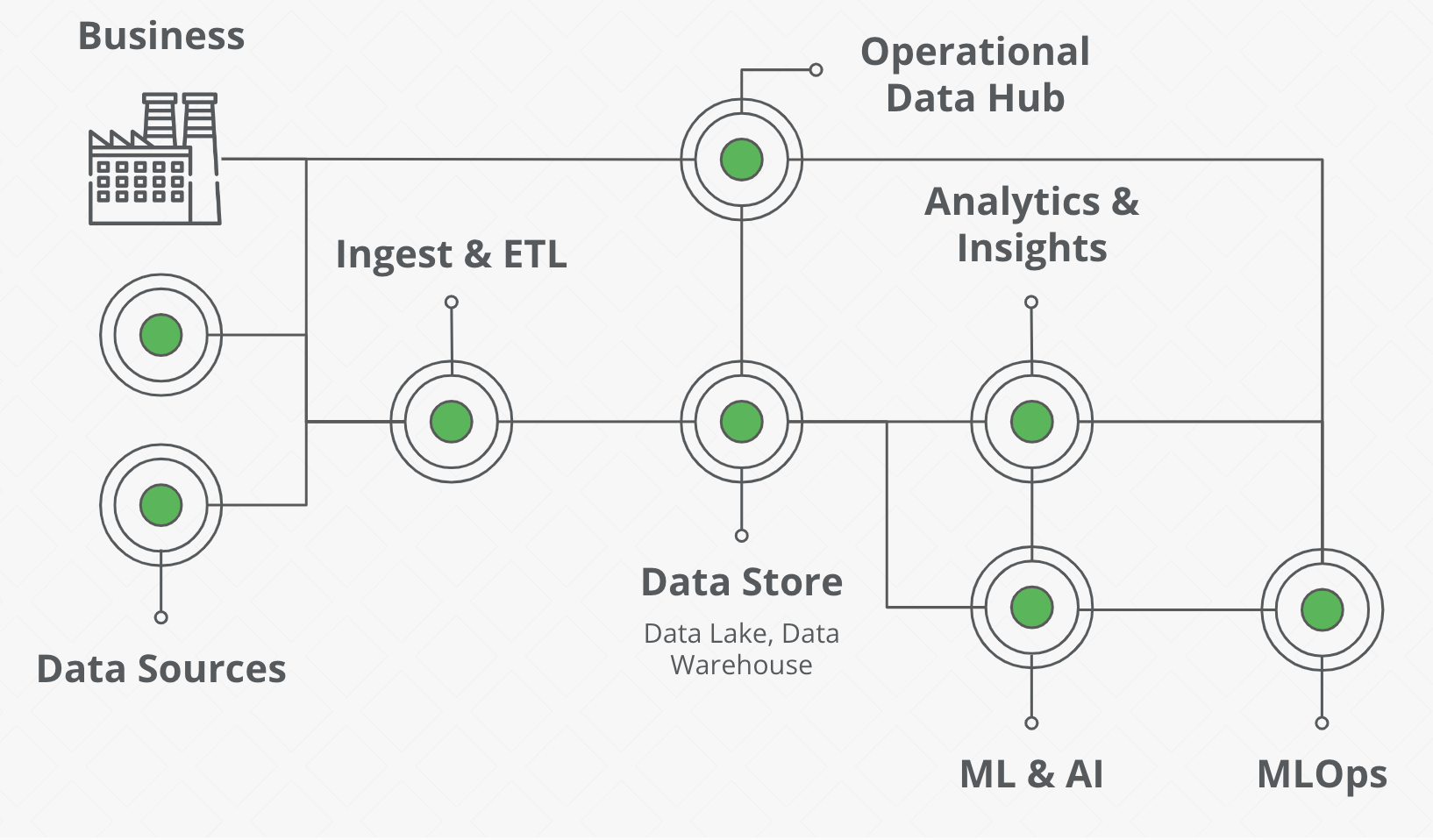
Solution: Invest in a platform to support the AI
Recognize the complexity and all of the people, money, and infrastructure that has to be invested in the AI platform. “If you go to ChatGPT right now, you’re one of thousands if not tens of thousands of people who are asking this AI model to generate text for you. So that requires distributed processing across a number of virtual machines and servers, and orchestrating all of that is important for users to even touch your tool,” said Brandon.
Of course, let’s not forget that building an AI system will require storage and utilization of a TON of data. “If you want to build AI, you need all of the data connected to the problem you want to solve,” said Brandon. Clients often talk about having so much data that their computer crashes when they open a spreadsheet, but that’s not enough. “If you have any concept of exactly how much data you have, then it’s not enough. AI requires ALL the data,” Brandon continued.
Investing in that infrastructure is money well spent, and it will prove critical to your AI implementation because “that infrastructure will outlive whatever you build.” The model will keep evolving and getting better, and what will remain is the underlying infrastructure.
Mistake #3: Viewing AI as self-sufficient
You can’t set it and forget it. Although many AI systems are considered self-learning, they still require consistent feedback to improve and grow. This idea of an unsupervised AI system may raise all kinds of societal and ethical concerns, but in reality, no AI system should be left unmonitored. You need to be connecting what you have in production back to analytics to monitor it and make sure it’s working. These AI analytics will provide the performance metrics on the system so that you understand overall usage (ex: volumes, success rates, trends, costs). On top of that, you also need to monitor and analyze the models actual outputs in process at the operational hub. This deeper level of analytics will ensure the model isn’t drifting over time, is providing valuable results, and generally continues to work as expected.
Solution: Consistently optimize the AI system
Optimization is really about deciding what success means and chasing that success. You need to set clear goals and establish KPIs to track the value the AI system is adding against the costs it incurs. The goal is for the implementation of AI to be better than the current state. Version 1 might not be better, but if it shows some value and has potential, you’ll probably move forward to get to Version 2. What if Version 2 isn’t better than the existing system? Is there enough value and potential to still move forward? What will you do if it’s never good enough? There’s no guarantee you’ll get to the performance you want and you should think about that upfront. Not all processes can go without human intervention. Knowing how to measure the value the AI system is creating and the costs it’s consuming will go a long way toward keeping forward momentum for the project and helping target enhancements for each iteration.
Mistake #4: Thinking all you need is data science
Data scientists are great at:
- Data collection and analysis
- Model development and testing
- Communication of results
But there are a long list of other tasks that need to happen with the implementation of an AI system, such as:
- Who manages and maintains the data resources?
- Who deploys the model and manages version control?
- Who supports and maintains the model in production?
- Who designs and builds the front-end UI?
- Who manages and controls access?
- Who is tracking, documenting, and resolving errors?
Solution: Identify and invest in the right talent
It’s not all Data Scientists. You will also need a team of people with skill sets that might include:
- Data Scientists
- DevOps Engineers
- MLOps or AIOps Engineers
- Data Engineers
- Full Stack Developers
- Digital Experience Researchers & Designers
“You need to ask questions like: What’s the structure of your data? What’s your cloud infrastructure look like? Where are all the systems? Do you have an ingestion pipeline?,” said Kelly. “And we quickly realize it isn’t just data scientists that we need. It’s a whole team of people from data engineers, DevOps engineers, MLOps engineers, digital experience designers, and full stack developers. Your data has to be curated and in a form that it can actually be ingested to add value to the problem set.”
One example that Brandon gave:
“This was a client that wanted to turn on ML. We said great! At that same time, that client had just released a network of thousands of IoT devices that were now in the field,” said Brandon. “So we said, well first thing we’re going to do is build you a streaming data architecture so that we can utilize all of this high-speed high-volume data coming from these IoT devices in real time. We had to build a whole new cloud architecture for them so they could take that data and utilize it to build their models. And a data scientist can’t do all that work for you, so we had to bring in data engineers and DevOps engineers to build that infrastructure for the data pipelines.” “It takes a village,” Kelly agreed.
If you don’t already have people with these skills, you can train up in these areas or add external resources to supplement capabilities. But this isn’t a one-time cost. “Once the model is built, the work is only beginning,” said Brandon. In production there will be a number of resources that need to be spun up to support the AI and distribute processing. “You don’t just write the check and you’re done. You have to keep paying for it - like kids,” joked Brandon. All of this comes with a cost and requires a team of people to support it.
AI is the big tech revolution we’re going to face - and the pace of change is only going to accelerate.
“When the car was being introduced and the current paradigm was the horse and buggy, everyone thought ‘what are we going to do with all our horses?’ Horses are fine. It’s just a new world. AI is a new paradigm.”
- Brandon Fischer
Ultimately though, if you’ve already started down the path of implementing AI, and suspect that you’ve made some of these mistakes, it’s not too late. Even if you didn’t spend six months developing an AI strategy, you can and should take a step back now and pull together that strategy. It will help you avoid other missteps in the future. Moving forward, you can steer and make corrections as you go along in a truly agile way.
How are you thinking about AI adoption in your business? Reach out - we’d love to chat.